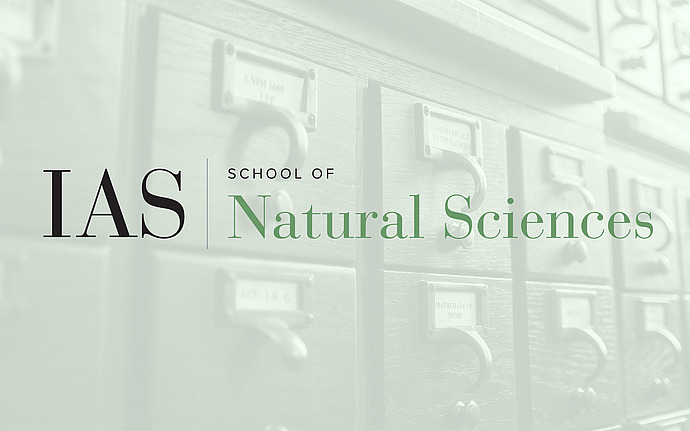
Princeton University Thunch Talk
Accelerated Bayesian Galaxy SED Modeling
Spectral Energy Distribution (SED) modeling is essential for galaxy evolution and cosmology to derive physical properties of galaxies, such as their stellar mass, star formation history, and chemical enrichment history, from observations. State-of-the-art SED modeling uses Bayesian inference, which requires sampling a high-dimensional parameter space. They take hundreds of CPU hours to analyze a single galaxy and, thus, are not scalable for the next-generation galaxy surveys. I will present how we can use new techniques in Machine Learning, such as neural emulation and neural density estimation, to dramatically accelerate Bayesian SED modeling. I will present how these techniques will be applied to the DESI Bright Galaxy Survey (BGS) to construct the PRObabilistic Value-Added BGS (PROVABGS), which will deliver full posteriors on the physical properties (e.g. stellar mass, star formation rate, metallicity, and age) of over 10 million galaxies out to z < 0.6. I will demonstrate the accuracy and precision of PROVABGS and discuss its scientific applications. Lastly, I will present new methods using neural posterior estimators that will accelerate Bayesian SED modeling even further and discuss their application to future surveys.