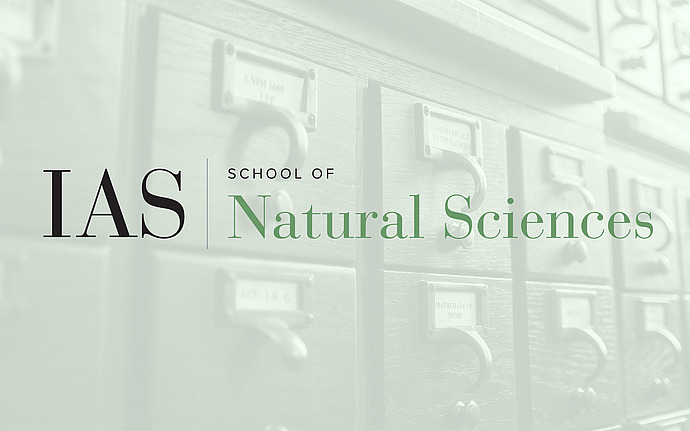
Princeton University Thunch Talk
Fast 2-body microlensing inference with neural posterior estimation
Owing to the pathological parameter space of binary microlensing which contains a multitude of local likelihood maximas that are narrow and deep, current analysis of such events are done on a case-by-case basis with computationally expensive “grid-searches” required as a prerequisite to MCMC posterior sampling. This status-quo approach creates a significant challenge on the scale of the Roman microlensing survey which is expected to discover thousands of such events. In this talk, I present a likelihood-free inference approach named neural posterior estimation, where a neural density estimator (NDE) learns a surrogate posterior as an observation-parameterized conditional probability distribution, from pre-computed simulations over the full prior space. After training on mere ~300,000 Roman-like 2L1S light-curve simulations, the NDE automatically produces accurate and precise posteriors for any future Roman light-curve within seconds, thus allowing for fast and automated inference. The NDE also captures expected posterior degeneracies.