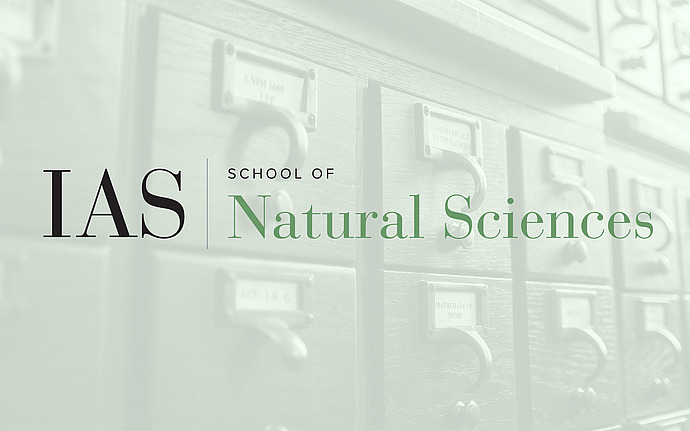
Rutgers University Astrophysics Seminar
Astronomy Re-envisioned: Investigating the Physics of Galaxy Evolution with Machine Learning
Machine learning (ML) techniques are revolutionizing our ability to study galaxy evolution and large-scale structure. Convolutional neural networks (CNNs) can now reliably predict galaxies' physical properties, including cold gas content and metallicity, directly from three-color optical images. These models can even reconstruct entire optical spectra from imaging alone. Highly optimized CNNs can also robustly identify nearby dwarf galaxies from wide-area surveys, significantly expanding the sample of known satellite systems at low redshifts. Meanwhile, graph neural networks (GNNs) can learn how large-scale environment impacts the galaxy-halo connection from cosmological simulations. These applications demonstrate how ML models with strong inductive biases can enable new scientific insights in galaxy evolution and cosmology. With upcoming wide-area surveys from the Vera C. Rubin Observatory and Nancy Grace Roman Space Telescope, advanced ML techniques will play an increasingly vital role in extracting physical understanding from vast cosmic datasets.