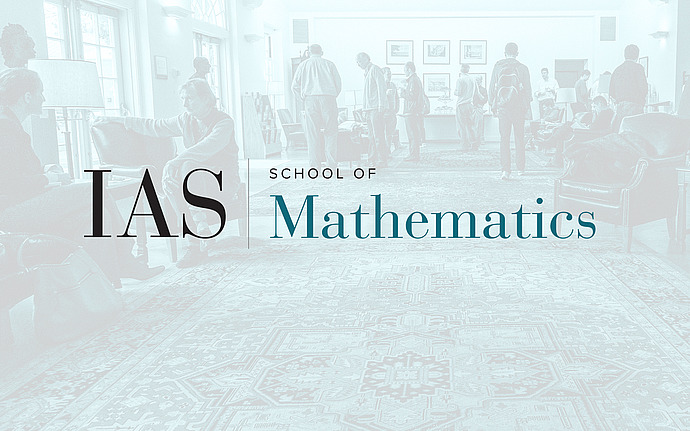
Computer Science/Discrete Mathematics Seminar I
Breaking \(e^n\) barrier for deterministic poly-time approximation of the permanent and settling Friedland's conjecture on the Monomer-Dimer Entropy
Two important breakthroughs on the permanent had been accomplished in 1998: A. Schrijver proved Schrijver-Valiant Conjecture on the minimal exponential growth of the number of perfect matchings in k-regular bipartite graphs with multiple edges; N. Linial, A. Samorodnitsky and A. Wigderson introduced a strongly poly-time deterministic algorithm to approximate the permanent of general non-negative matrices within the multiplicative factor en. Many things happened since them, notably the prize-winning Jerrum, Vigoda, Sinclair FPRAS for the permanent. Schrijver's lower bound was vastly generalized and improved; moreover the new proofs, based on hyperbolic(aka stable) polynomials, are transparent, easy, non-computational. Yet, until now there were no deterministic poly- time algorithms to approximate the permanent of general non-negative matrices within the multiplicative factor \(F^n\), \(F < e\). We prove the following double-sided inequality for doubly-stochastic matrices \(A\): \[ \prod_{1 \leq i,j \leq n} (1 - A(i,j))^{1-A(i,j)} \leq per(A) \leq C^n \prod_{1 \leq i,j \leq n} (1 - A(i,j))^{1-A(i,j)},\] where \(C \approx 1.9022\). Thus a simple(of linear complexity) extension of the scaling algorithm approximates the permanent within the multiplicative factor \( \approx 1.9022^n\). A slightly more involved argument proves S. Friedland's conjecture on the Monomer-Dimer Entropy, which is a generalization of Schrijver-Valiant Conjecture to partial matchings(aka dimers) that in the limit cover a given fraction \(t \in [0, 1]\) of vertices. The main result in this direction is asymptotically sharp lower bounds on the coefficients of the weighted matching polynomial associated with a doubly-stochastic matrix. We note that such polynomials played crucial role in the recent breakthrough on the existence of “large” bipartite regular Ramunajan Graphs with prescribed degree. The talk is for general mathematical/computer science/physics audience. I will gently go through the very rich and surprising history of the topic: amazingly, the popular statistical physics(and lately machine learning) heuristic, called Bethe Approximation, is one of the (completely rigorous) keys in our approach. The Bethe Approximation was already applied by physicists to the Monomer-Dimer Problem in late 1930s and was mysteriously hidden in Schrijver’s 1998 paper. Time permitting, a conjecture, connecting the Bethe Approximation, Correlation Inequalities and hyperbolic polynomials, will be stated and discussed. (Joint work with Alex Samorodnitsky (Hebrew University).)