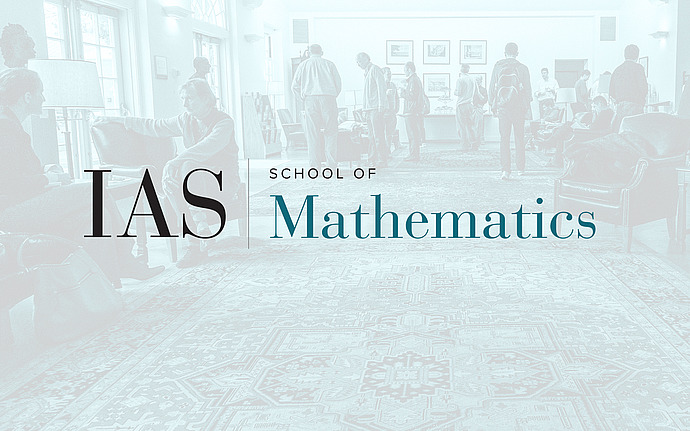
Workshop on Machine Learning, Theory, and Method in the Social Sciences
The workshop was by invitation-only.
Can the dramatic recent progress in machine learning lead to conceptual advances in the social sciences and humanities? Although we have access to social and cultural datasets of unprecedented scale, quality, and complexity, this question remains open. New methods of machine learning (e.g., various flavors of deep nets, transformer nets, AlphaGo and the like) often take a "black box" view of data. Little theoretical understanding exists about what patterns in data were implicitly identified as part of the learning.
But it is these patterns that are of primary interest in the social sciences, especially when researchers hope to discover new social processes or phenomena.
This workshop aimed to bridge the gap via focused dialog between the two communities. ML experts learned about questions in culture, cognition, social action, power relations etc. that might inform the design of ML systems in the laboratory and “in the wild.” Social scientists arrived at a better understanding of how modern ML techniques might be leveraged to generate new research projects and transformative methodological innovations. Out-of-the-box talks and discussions were highly encouraged!
Organizers:
The workshop was organized by Sanjeev Arora (IAS/Princeton University), Didier Fassin (IAS), Jacob Foster (UCLA), and Marion Fourcade (UC Berkeley/IAS)
Confirmed participants:
Elizabeth Bruch, Cristian Danescu-Niculescu-Mizil, James Evans, Filiz Garip, Tom Griffiths, Justin Grimmer, Zubin Jelveh, Monica Lee, Alondra Nelson, Etienne Ollion, Matt Salganick, Brandon Stewart, Diyi Yang
Agenda (by invitation only)
Slides for talks:
Public Lecture: 3/4 at 5:30 pm in Wolfensohn Hall
Elizabeth Bruch, University of Michigan, "Discovering Decision Rules in Mate Choice"
Thomas Griffiths, Princeton University, "Human Behavior as the Next Fronteir for AI"
Video: https://youtu.be/AMNzZkZZY2A