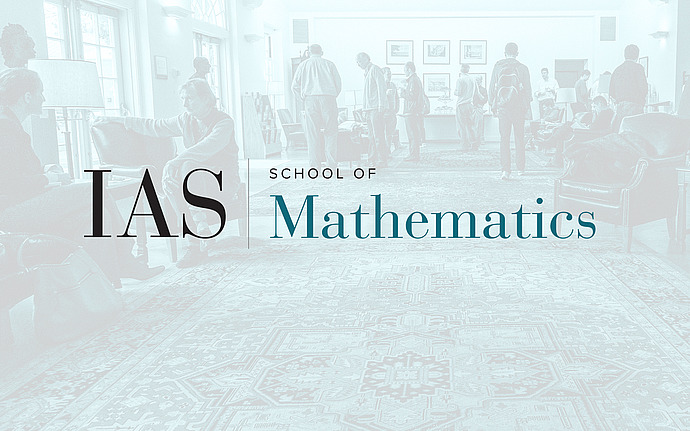
Computer Science/Discrete Mathematics Seminar I
Private Optimization and Statistical Physics: Low-Rank Matrix Approximation
In this talk, I will present the following two connections between private optimization and statistical physics, both via the problem of approximating a given covariance matrix with a low-rank matrix:
- An efficient algorithm to privately compute a low-rank approximation to a given matrix and how it leads to an efficient way to sample from Harish-Chandra-Itzykson-Zuber densities studied in physics and mathematics. (Based on https://arxiv.org/abs/2011.05417)
- A new way to analyze the "utility" of the "Gaussian Mechanism" for private low-rank approximation using Dyson Brownian motion and how it leads to new eigenvalue gap bounds for random matrices. (Based on https://arxiv.org/abs/2211.06418 and https://arxiv.org/abs/2306.16648)
Date & Time
October 09, 2023 | 11:15am – 12:15pm
Location
Simonyi 101 and Remote AccessSpeakers
Nisheeth Vishnoi, Yale University