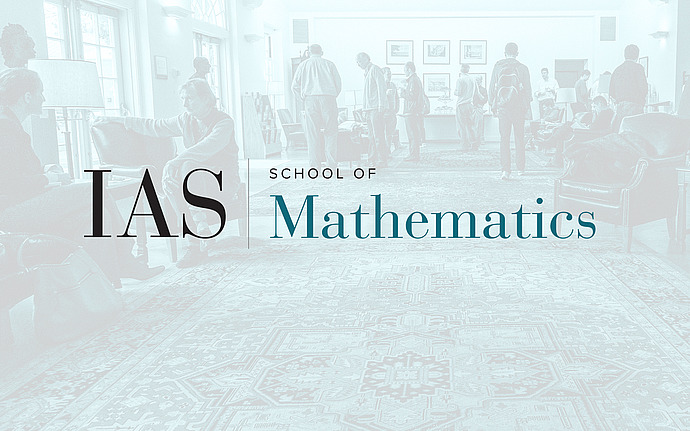
Computer Science/Discrete Mathematics Seminar II
Extending Generalization Theory to Address Phenomena in Contemporary Machine Learning
Recent years have seen remarkable progress in the field of Machine Learning (ML).
However recent breakthroughs exhibit phenomena that remain unexplained and at times contradict conventional wisdom. A primary reason for this discrepancy is that classical ML theory adopts a worst-case perspective which often appears overly pessimistic for explaining practical ML scenarios. In reality data is rarely worst-case and experimental evidence suggests that often much less data is needed than predicted by traditional theory.
In this tutorial we will overview the classical Vapnik-Chervonenkis Theory along with two variations that offer distribution- and data-dependent perspectives. These variations complement the classical theory's worst-case (distribution-free) perspective and are well-suited for leveraging specific properties of a given learning task. A unifying theme among these models is their combinatorial nature, marking a continuation of the relationship between machine learning and combinatorics—a connection dating back to the discovery of the VC dimension over 50 years ago.