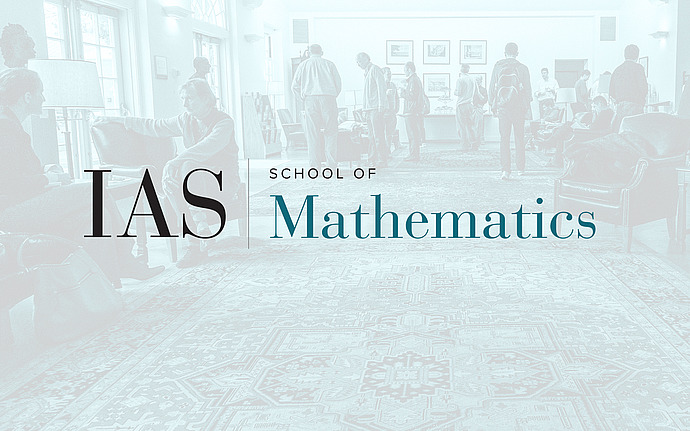
Computer Science/Discrete Mathematics Seminar I
Optimal Weak to Strong Learning
The classic algorithm AdaBoost allows to convert a weak learner, that is an algorithm that produces a hypothesis which is slightly better than chance, into a strong learner, achieving arbitrarily high accuracy when given enough training data. We present a new algorithm that constructs a strong learner from a weak learner but uses less training data than AdaBoost and all other weak to strong learners to achieve the same generalization bounds. A sample complexity lower bound shows that our new algorithm uses the minimum possible amount of training data and is thus optimal. Hence, this work settles the sample complexity of the classic problem of constructing a strong learner from a weak learner.
This work was accepted at NeurIPS’22 and is joint work with Martin Ritzert from Aarhus University