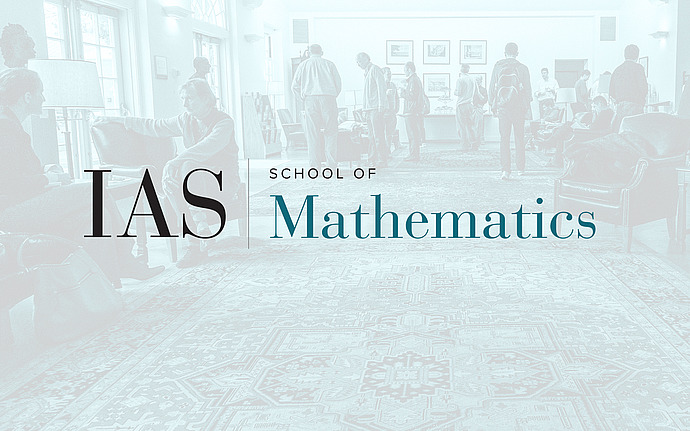
Computer Science/Discrete Mathematics Seminar II
Localization schemes: A framework for proving mixing bounds for Markov chains
Two recent and seemingly-unrelated techniques for proving mixing bounds for Markov chains are:
(i) the framework of Spectral Independence, introduced by Anari, Liu and Oveis Gharan, and its numerous extensions, which have given rise to several breakthroughs in the analysis of mixing times of discrete Markov chains and
(ii) the Stochastic Localization technique which has proven useful in establishing mixing and expansion bounds for both log-concave measures and for measures on the discrete hypercube.
We will present a framework which connects ideas from both techniques and by doing so unifies, simplifies and extends those techniques, providing an approach that works in both discrete and continuous settings. In its center is the concept of a ``localization scheme'' which, to every probability measure on some space $\Omega$ (which will usually be either the discrete hypercube or $R^n$, assigns a martingale of probability measures which ``localize'' in space as time evolves. As it turns out, to every such scheme corresponds a Markov chain, and many chains of interest appear naturally in this framework. This viewpoint provides tools for deriving mixing bounds for the dynamics through the analysis of the corresponding localization process. Generalizations of concepts of Spectral Independence and Entropic Independence naturally arise from our definitions, and in particular we will show how to recover the main theorems in the spectral and entropic independence frameworks via simple martingale arguments (completely bypassing the need to use the theory of high-dimensional expanders). We demonstrate how to apply our machinery towards simple proofs to many mixing bounds in the recent literature. In particular, we will discuss how to use it to obtain the first $O(n \log n)$ bound for mixing time of the hardcore-model (of arbitrary degree) in the tree-uniqueness regime, under Glauber dynamics and to prove a KL-divergence decay bound for log-concave sampling via the Restricted Gaussian Oracle, which achieves optimal mixing under any $\exp\left (n\right )$-warm start.
Based on a joint work with Yuansi Chen.