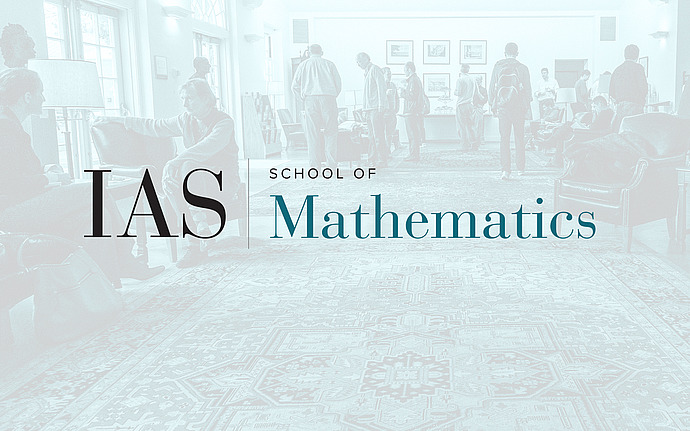
Computer Science/Discrete Mathematics Seminar I
PAC Learnability of partial concept classes
We extend the classical theory of PAC learning in a way which allows to model a rich variety of practical learning tasks where the data satisfies special properties that ease the learning process. This is done by considering partial concepts: functions that can be undefined on certain parts of the space, providing a natural way to express assumptions on the data such as satisfying margin conditions.
We characterize PAC learnability of partial concept classes and reveal an algorithmic landscape which is fundamentally different than the classical one. We also show that there are easy-to-learn partial concept classes which probably cannot be captured by the traditional PAC theory, resolving in a strong sense a recent problem of Attias, Kontorovich and Mansour.
Joint work with Steve Hanneke, Ron Holzman and Shay Moran.