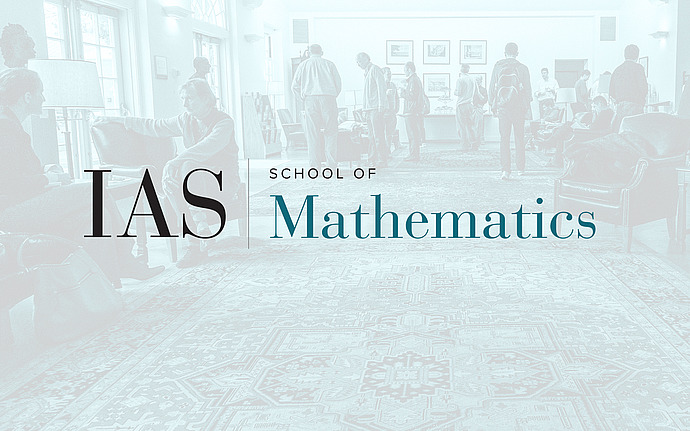
Computer Science/Discrete Mathematics Seminar II
Computational - Statistical gaps and the Group Testing problem
In a plethora of random models for constraint satisfaction and statistical inference problems, researchers from different communities have observed computational gaps between what existential or brute-force methods promise, and what known efficient algorithms achieve. In this talk, I will discuss this phenomenon in the context of the so-called Group Testing problem.
Group Testing is a fundamental problem in statistical inference with many real-world applications. The goal is to detect a set of k defective items out of a population of size p using n << p tests. Towards that end, one is allowed to utilize a procedure that can test groups of items, in which each test is returned positive if and only if at least one item in the group is defective. In this talk, I will discuss the task of approximate recovery, in which we tolerate having a small number of incorrectly classified items.