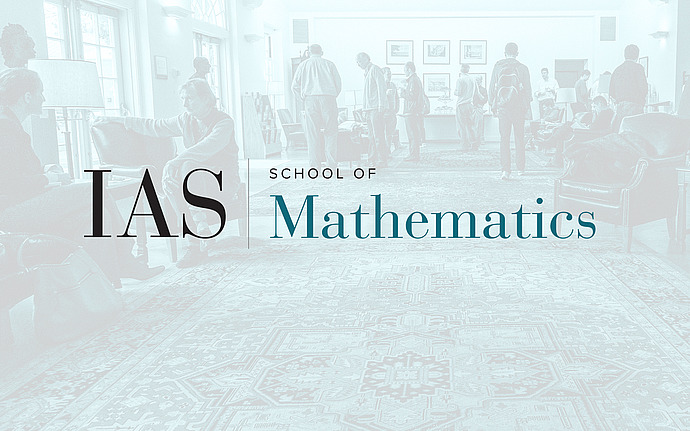
Theoretical Machine Learning Seminar
A Blueprint of Standardized and Composable Machine Learning
In handling wide range of experiences ranging from data instances, knowledge, constraints, to rewards, adversaries, and lifelong interplay in an ever-growing spectrum of tasks, contemporary ML/AI research has resulted in thousands of models, learning paradigms, optimization algorithms, not mentioning countless approximation heuristics, tuning tricks, and black-box oracles, plus combinations of all above. While pushing the field forward rapidly, these results also make a comprehensive grasp of existing ML techniques more and more difficult, and make standardized, reusable, repeatable, reliable, and explainable practice and further development of ML/AI products quite costly, if possible, at all. In this talk, we present a simple and systematic blueprint of ML, from the aspects of losses, optimization solvers, and model architectures, that provides a unified mathematical formulation for learning with all experiences and tasks. The blueprint offers a holistic understanding of the diverse ML algorithms, guidance of operationalizing ML for creating problem solutions in a composable and mechanic manner, and unified framework for theoretical analysis.
Date & Time
Location
Remote Access Only - see link belowSpeakers
Affiliation
Event Series
Categories
Notes
We welcome broad participation in our seminar series. To receive login details, interested participants will need to fill out a registration form accessible from the link below. Upcoming seminars in this series can be found here.