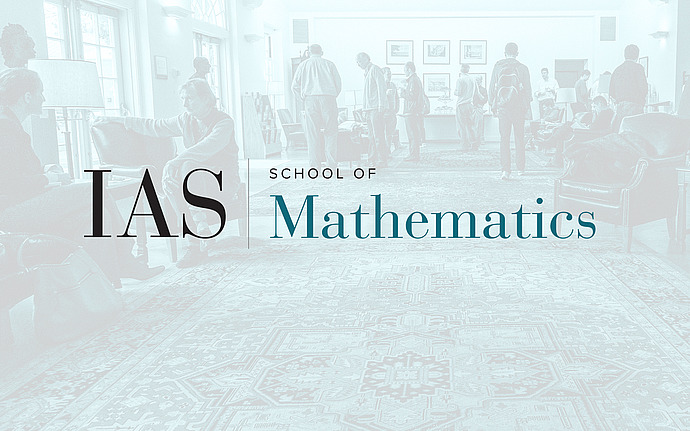
Theoretical Machine Learning Seminar
The challenges of model-based reinforcement learning and how to overcome them
Some believe that truly effective and efficient reinforcement learning algorithms must explicitly construct and explicitly reason with models that capture the causal structure of the world. In short, model-based reinforcement learning is not optional. As this is not a new belief, it may be surprising that empirically, at least as far as the current state of art is concerned, the majority of the top performing algorithms are model-free. In this talk, I will define three major challenges that need to be overcome for model-based methods to take their place above, or before the model-free ones: (1) planning with large models; (2) models are never well-specified; (3) models need to focus on task relevant aspects and ignore others. For each of the challenges, I will describe recent results that address them and I will also take a tally of the most interesting (and challenging) remaining open problems.
Date & Time
Location
Remote Access Only - see link belowSpeakers
Affiliation
Event Series
Categories
Notes
We welcome broad participation in our seminar series. To receive login details, interested participants will need to fill out a registration form accessible from the link below. Upcoming seminars in this series can be found here.