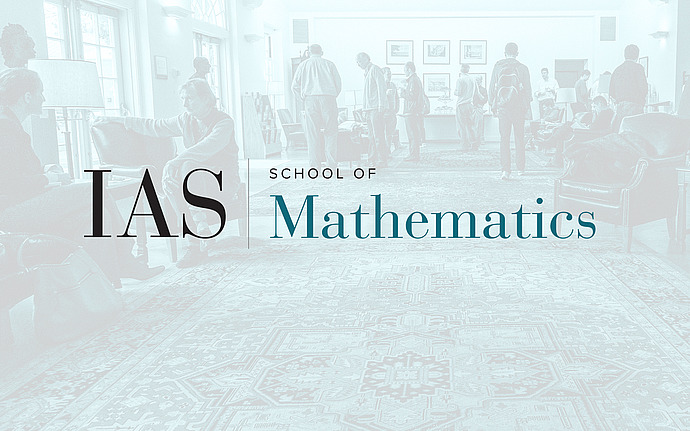
Workshop on New Directions in Optimization, Statistics and Machine Learning
The Peculiar Optimization and Regularization Challenges in Multi-Task Learning and Meta-Learning
Despite the success of deep learning, much of its success has existed in settings where the goal is to learn one, single-purpose function from data. However, in many contexts, we hope to optimize neural networks for multiple, distinct tasks (i.e. multi-task learning), and optimize so that what is learned from these tasks is transferable to the acquisition of new tasks (e.g. as in meta-learning). In this talk, I will discuss how the multi-task and meta optimization problems differ from standard problems, and some of the unique challenges that arise, both in optimization and in regularization. This includes (1) a kind of overfitting that is unique to meta-learning, where the optimizer memorizes not the labels, but the functions that solve the training tasks; and (2) challenging optimization landscapes that are common in multi-task learning settings. In both cases, I will present concrete characterizations of the underlying problems, and steps we can take to mitigate them. By taking these steps, we observe substantial gains in practice.