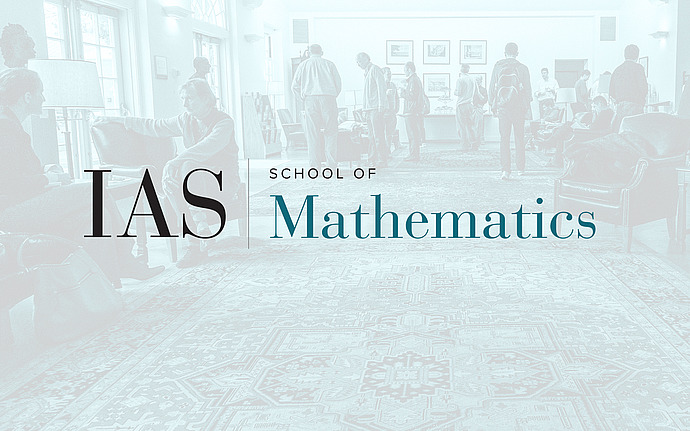
Workshop on New Directions in Optimization, Statistics and Machine Learning
Do Simpler Models Exist and How Can We Find Them?
While the trend in machine learning has tended towards more complex hypothesis spaces, it is not clear that this extra complexity is always necessary or helpful for many domains. In particular, models and their predictions are often made easier to understand by adding interpretability constraints. These constraints shrink the hypothesis space; that is, they make the model simpler. Statistical learning theory suggests that generalization may be improved as a result as well. However, adding extra constraints can make optimization (exponentially) harder. For instance it is much easier in practice to create an accurate neural network than an accurate and sparse decision tree. We address the following question: Can we show that a simple-but-accurate machine learning model might exist for our problem, before actually finding it? If the answer is promising, it would then be worthwhile to solve the harder constrained optimization problem to find such a model. In this talk, I present an easy calculation to check for the possibility of a simpler model. This calculation indicates that simpler-but-accurate models do exist in practice more often than you might think. Time-permitting, I will then briefly overview our progress towards the challenging problem of finding optimal sparse decision trees.
This is joint work with Lesia Semenova, Ron Parr, Xiyang Hu, Chudi Zhong, Jimmy Lin, and Margo Seltzer.