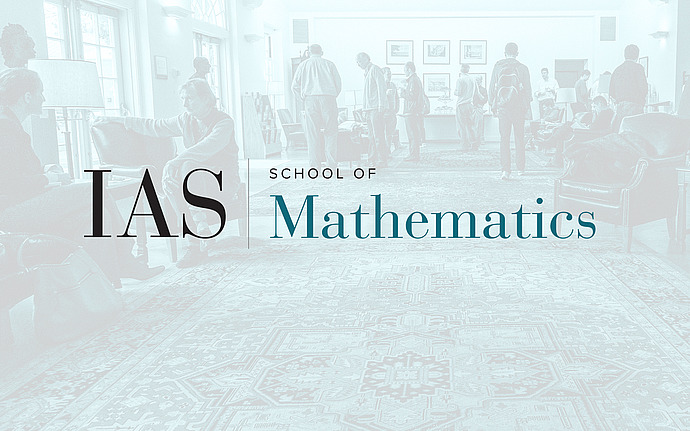
Theoretical Machine Learning Seminar
Latent Stochastic Differential Equations for Irregularly-Sampled Time Series
Much real-world data is sampled at irregular intervals, but most time series models require regularly-sampled data. Continuous-time models address this problem, but until now only deterministic (ODE) models or linear-Gaussian models were efficiently trainable with millions of parameters. We construct a scalable algorithm for computing gradients of samples from stochastic differential equations (SDEs), and for gradient-based stochastic variational inference in function space, all with the use of adaptive black-box SDE solvers. This allows us to fit a new family of richly-parameterized distributions over time series. We apply latent SDEs to motion capture data, and will also discuss prototypes of infinitely-deep Bayesian neural networks.
Date & Time
Location
Remote Access Only - see link belowSpeakers
Affiliation
Event Series
Categories
Notes
Please note: interested participants will need to fill out this google form in advance to obtain access to this seminar. Once approved, you will receive the login details. Members of the IAS community do not need to fill out this form - the login details will be emailed to you.
https://forms.gle/vxPMgdiURWpRqrV8A