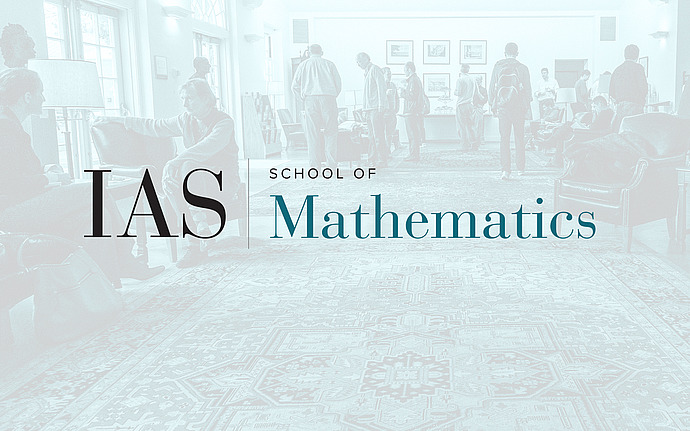
Theoretical Machine Learning Seminar
Preference Modeling with Context-Dependent Salient Features
This talk considers the preference modeling problem and addresses the fact that pairwise comparison data often reflects irrational choice, e.g. intransitivity. Our key observation is that two items compared in isolation from other items may be compared based on only a salient subset of features. Formalizing this idea, I will introduce our proposal for a “salient feature preference model” and discuss sample complexity results for learning the parameters of our model and the underlying ranking with maximum likelihood estimation. I will also provide empirical results that support our theoretical bounds, illustrate how our model explains systematic intransitivity, and show in this setting that our model is able to recover both pairwise comparisons and rankings for unseen pairs or items. Finally I will share results on two data sets: the UT Zappos50K data set and comparison data about the compactness of legislative districts in the US. This is joint work with Amanda Bower at the University of Michigan.