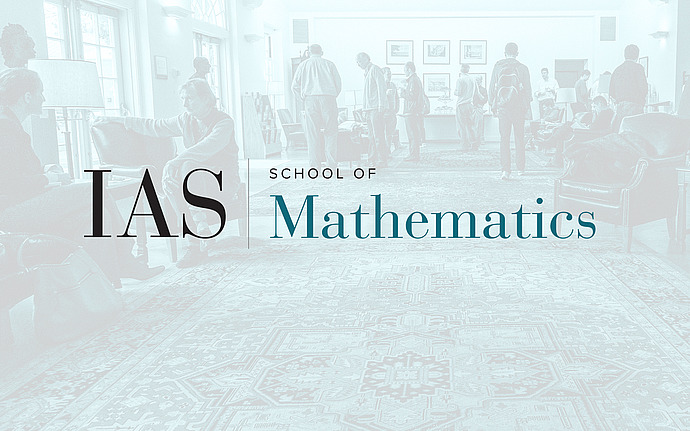
Theoretical Machine Learning Seminar
The Lottery Ticket Hypothesis: On Sparse, Trainable Neural Networks
We recently proposed the "Lottery Ticket Hypothesis," which conjectures that the dense neural networks we typically train have much smaller subnetworks capable of training in isolation to the same accuracy starting from the original initialization. This hypothesis raises questions about the nature of overparameterization and the importance of initialization for training neural networks in practice. In this talk, I will discuss existing work and the latest developments on the "Lottery Ticket Hypothesis," including the empirical evidence for these claims on small vision tasks, changes necessary to scale these ideas to ImageNet, and the relationship between these subnetworks and their "stability" to the noise of stochastic gradient descent. This research is entirely empirical, although it has exciting implications for theory. (This is joint work with Gintare Karolina Dziugaite, Daniel M. Roy, Michael Carbin, and Alex Renda.)