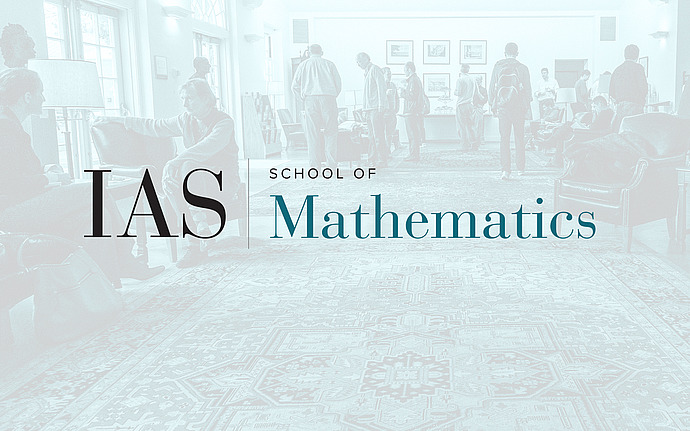
Joint IAS/Princeton University Theoretical Machine Learning Seminar
Can learning theory resist deep learning?
Machine learning algorithms are ubiquitous in most scientific, industrial and personal domains, with many successful applications. As a scientific field, machine learning has always been characterized by the constant exchanges between theory and practice, with a stream of algorithms that exhibit both good empirical performance on real-world problems and some form of theoretical guarantees. Many of the recent and well publicized applications come from deep learning, where these exchanges are harder to make, in part because the objective functions used to train neural networks are not convex. In this talk, I will present recent results on the global convergence of gradient descent for some specific non-convex optimization problems, illustrating these difficulties and the associated pitfalls (joint work with Lénaïc Chizat and Edouard Oyallon).