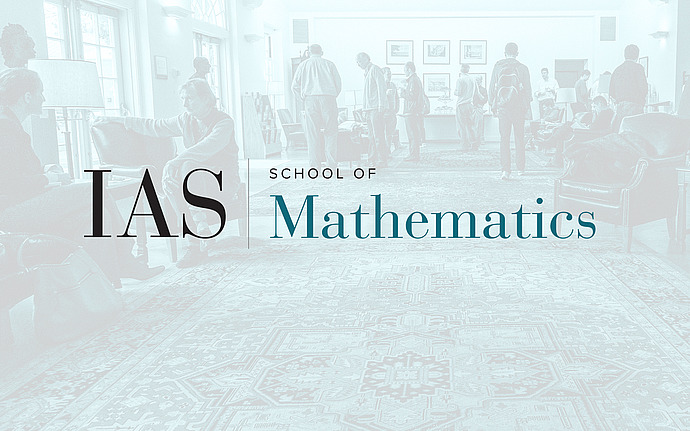
Theoretical Machine Learning Seminar
Towards a better understanding of neural networks: learning dynamics, interpretability and RL generalization
With the continuing successes of deep learning, it becomes increasingly important to better understand the phenomena exhibited by these models, ideally through a combination of systematic experiments and theory. In this talk I discuss some of our work addressing questions in this space. In the first part, I describe adapting Canonical Correlation Analysis (SVCCA), as a tool for quickly comparing two representations in a way that is both invariant to affine transform (allowing comparison between different layers and networks) and fast to compute (allowing more comparisons to be calculated than with previous methods). We deploy this tool to measure the intrinsic dimensionality of layers, showing in some cases needless over-parameterization; to probe learning dynamics throughout training, finding that networks converge to final representations from the bottom up; to show where class-specific information in networks is formed; and to suggest new training regimes that simultaneously save computation and overfit less. In the second part, I overview a new testbed of environments for deep reinforcement learning that let us study different RL algorithms, compare to supervised learning, and evaluate generalization in a systematic way.