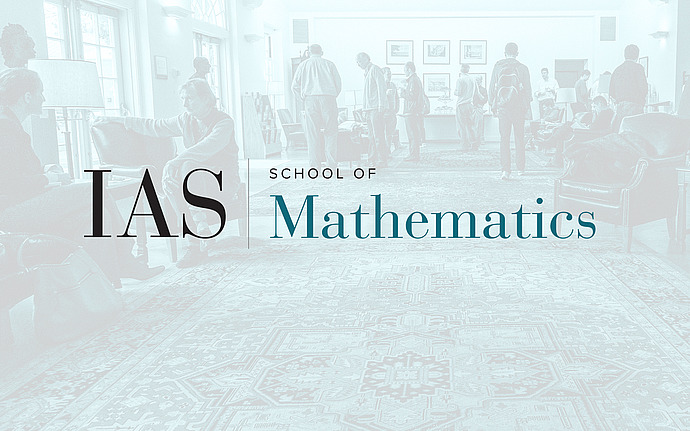
Workshop on New Directions in Optimization, Statistics and Machine Learning
A snapshot of few-shot classification
Few-shot classification, the task of adapting a classifier to unseen classes given a small labeled dataset, is an important step on the path toward human-like machine learning. I will present some of the key advances in this area, and will then focus on the fundamental issue of overfitting in the few-shot scenario. Bayesian methods are well-suited to tackling this issue because they allow practitioners to specify prior beliefs and update those beliefs in light of observed data. Contemporary approaches to Bayesian few-shot classification maintain a posterior distribution over model parameters, which is slow and requires storage that scales with model size. Instead, we propose a Gaussian process classifier based on a novel combination of Pólya-gamma augmentation and the one-vs-each loss that allows us to efficiently marginalize over functions rather than model parameters. We demonstrate improved accuracy and uncertainty quantification on both standard few-shot classification benchmarks and few-shot domain transfer tasks. I will conclude by discussing open questions in this area.