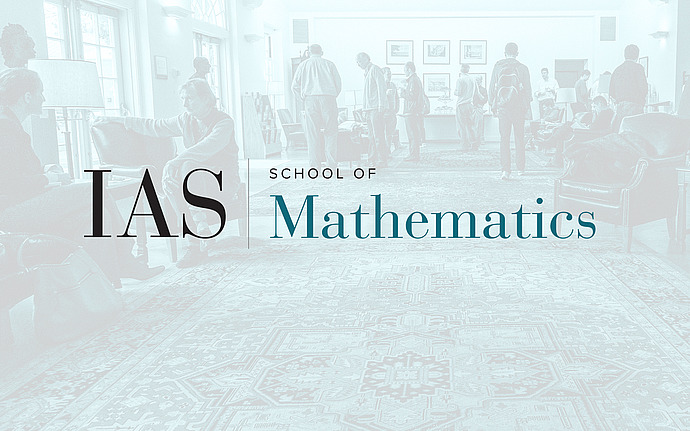
Virtual Workshop on Missing Data Challenges in Computation, Statistics and Applications
Gene expression recovery in single cell transcriptomic data
Abstract: Cells are the basic biological units of multicellular organisms. The development of single-cell technologies such as single cell RNA sequencing (scRNA-seq) have enabled us to study the diversity of cell types in tissue and to elucidate the roles of individual cell types in disease. Single cell RNA-seq data are noisy and sparse. The efficiency, that is, the proportion of transcripts in the cell that are eventually counted, can vary between 2-60%, and can be especially low in highly parallelized technologies. This leads to a severe case of not-at-random missing data, which hinders and confounds analysis, especially for low to moderately expressed genes. In this talk, I will describe, SAVER, a noise reduction and missing-data imputation framework for single cell RNA sequencing. We illustrate how this critical recovery step allows improves cell-type classification, increased power in the identification of cell type markers, and more accurate assessment of gene-gene relationships at the single cell level. I will also describe a transfer learning framework based on deep neural nets to borrow information across related single cell data sets for de-noising. Our goal is to leverage the expanding resources of publicly available scRNA-seq data, for example, the Human Cell Atlas which aims to be a comprehensive map of cell types in the human body. Through this framework, we explore the limits of data sharing: How much can be learned across cell types, tissues, and species? How useful are data from other technologies and labs in improving the estimates from your own study? If time allows, I will also discuss the implications of such data denoising to downstream statistical inference.