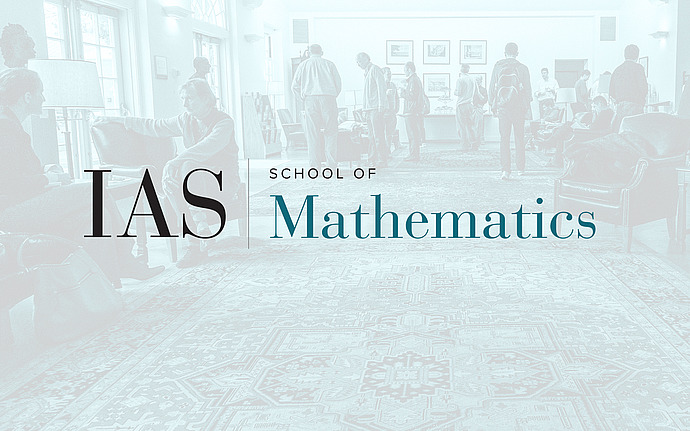
Virtual Workshop on Missing Data Challenges in Computation, Statistics and Applications
Model-based clustering of high-dimensional data: Pitfalls & solutions
Abstract: In many applications, it is of interest to cluster subjects based on very high-dimensional data, often in the presence of missing data. Although discrete mixture models are routinely used, we demonstrate pitfalls in high-dimensional settings. As we are interested in characterizing uncertainty in clustering, we focus on Bayesian methods. As the dimension p increases, we find that (1) MCMC mixing gets worse and worse; and (2) the true posterior often has aberrant limiting behavior, assigning all observations to the same cluster or to different clusters. We propose LAtent Mixtures for Bayesian (Lamb) clustering to solve (1)-(2) by clustering based on a low-dimensional latent variable. We provide theoretical support showing that the posterior over partitions approximates the posterior obtained by an oracle having knowledge of a lower-dimensional representation of the data. Substantial gains relative to competitors are shown in simulations and the methods are applied to clustering of single cell RNAseq data. The methods can trivially handle missing data under MAR assumptions.
Joint work with Noirrit Kiran Chandra & Tony Canale