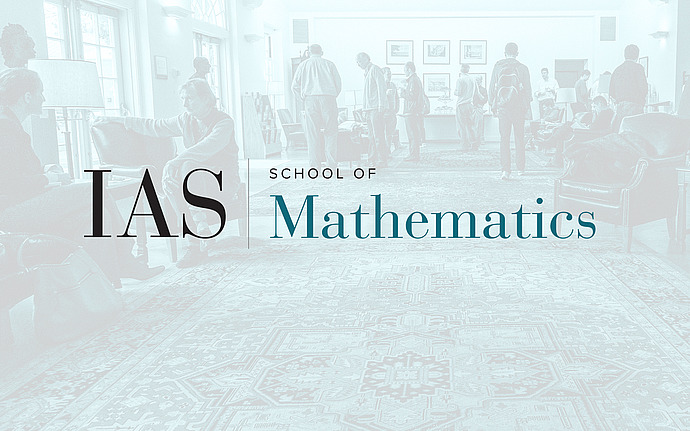
Virtual Workshop on Missing Data Challenges in Computation, Statistics and Applications
High-dimensional omics data analysis with missing values
Abstract: We have seen the rise of high-dimensional omics data, e.g., genome, transcriptome, microbiome, and proteome in recent decades. The different types of missingness in modern omics data bring up significant biological and statistical challenges. In this talk, we focus on two problems in modern omics data analysis with missing values.
First, motivated by applications in genomic data integration, we propose a new framework of structured matrix completion (SMC) that treats structured missingness by design. Specifically, our proposed method aims at efficient matrix recovery when a subset of the rows and columns of an approximately low-rank matrix are observed. We provide theoretical justification for the proposed method and derive lower bound for the estimation errors, which together establish the optimal rate of recovery over certain classes of approximately low-rank matrices. The method is applied to integrate several ovarian cancer genomic studies with different extent of genomic measurements, which enables us to construct more accurate prediction rules for ovarian cancer survival.
Then, we address the common issues of missing counts and the high variability in the sequencing reads in the microbiome data. We introduce a surprisingly simple, interpretable, and efficient method for the estimation of compositional data regression through the lens of a novel high-dimensional log-error-in-variable regression model. The proposed method provides both corrections on sequencing data with possible overdispersion and simultaneously avoids any subjective imputation of missing read counts. We provide theoretical justification with matching upper and lower bounds for the estimation error. The merit of the procedure is illustrated through real microbiome data analysis and simulation studies.