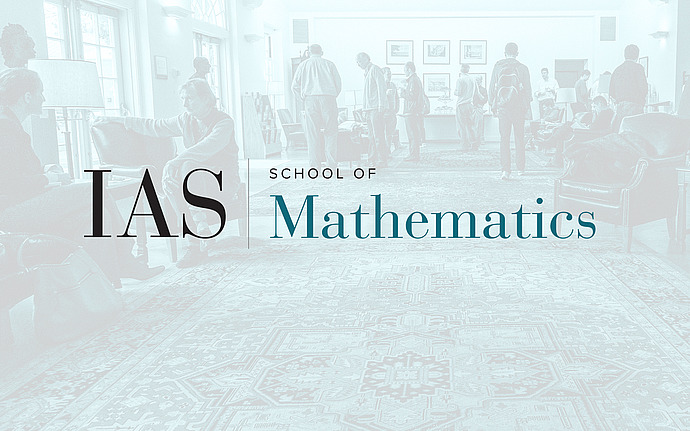
Theoretical Machine Learning Seminar - PCTS Seminar Series: Deep Learning for Physics
Dynamics of Generalization in Overparameterized Neural Networks
Please Note: The seminars are not open to the general public, but only to active researchers.
Register here for this event: https://docs.google.com/forms/d/e/1FAIpQLScJ-BUVgJod6NGrreI26pedg8wGEyPhh3WMDskE1hIac_Yp3Q/viewform
What interplay of dynamics, architecture, and data make good generalization possible in overparameterized neural networks? Approaches from statistical physics have shed light on this question by considering a variety of simple limiting cases. I will describe results emerging from two simple models: deep linear neural networks and nonlinear student-teacher networks. In these models, good generalization from limited data arises from aspects of training dynamics and initialization. Finally, I will briefly tour open problems facing practitioners that seem amenable to analysis with similar methods.
Date & Time
Location
Jadwin Hall PCTS Seminar Room 407 (Princeton University)Speakers
Affiliation
Categories
Notes
Each talk will be preceded with lunch at 11:45 am. The talks will be held from 12:25-1:30 pm and from 2:00 - 3:00 pm.