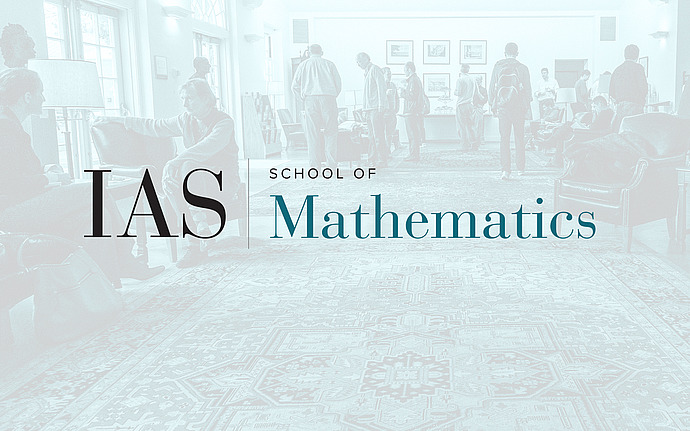
Theoretical Machine Learning Seminar
What Do Our Models Learn?
Large-scale vision benchmarks have driven---and often even defined---progress in machine learning. However, these benchmarks are merely proxies for the real-world tasks we actually care about. How well do our benchmarks capture such tasks?
In this talk, I will discuss the alignment between our benchmark-driven ML paradigm and the real-world uses cases that motivate it. First, we will explore examples of biases in the ImageNet dataset, and how state-of-the-art models exploit them. We will then demonstrate how these biases arise as a result of design choices in the data collection and curation processes.
Based on joint works with Logan Engstrom, Andrew Ilyas, Shibani Santurkar, Jacob Steinhardt, Dimitris Tsipras and Kai Xiao.
Date & Time
Location
Remote Access Only - see link belowSpeakers
Affiliation
Event Series
Categories
Notes
We welcome broad participation in our seminar series. To receive login details, interested participants will need to fill out a registration form accessible from the link below. Upcoming seminars in this series can be found here.