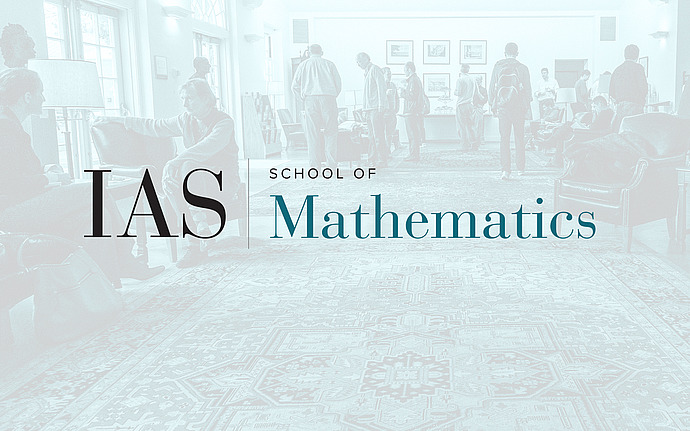
Theoretical Machine Learning Seminar
Learning Controllable Representations
As deep learning systems become more prevalent in real-world applications it is essential to allow users to exert more control over the system. Exerting some structure over the learned representations enables users to manipulate, interpret, and even obfuscate the representations, and may also improve out-of-distribution generalization. In this talk I will discuss recent work that makes some steps towards these goals, aiming to represent the input in a factorized form, with dimensions of the latent space partitioned into task-dependent and task-independent components. I will focus on an approach that utilizes a reversible formulation of a network. Doing this reveals a lack of robustness: the system is too invariant to a wide range of task-relevant changes, leading to a novel form of adversarial attacks which we term excessive invariance. Our main contribution is an information-theoretic objective that encourages the model to develop factorized representations. This provides the first approach tailored explicitly to overcome excessive invariance and resulting vulnerabilities. It also facilitates several applications, including domain adaptation.