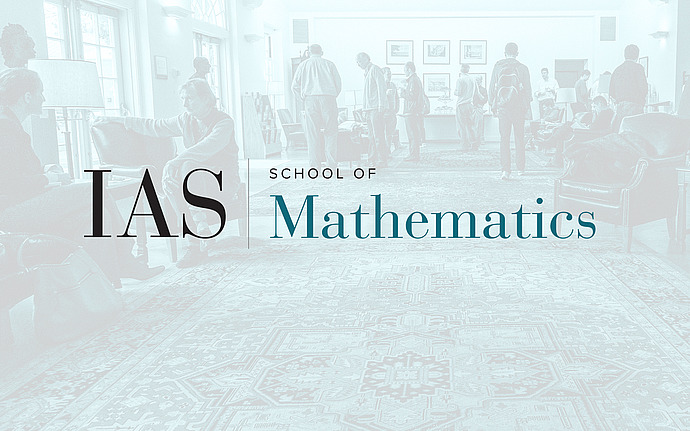
Theoretical Machine Learning Seminar
Margins, perceptrons, and deep networks
This talk surveys the role of margins in the analysis of deep networks. As a concrete highlight, it sketches a perceptron-based analysis establishing that shallow ReLU networks can achieve small test error even when they are quite narrow, sometimes even logarithmic in the sample size and inverse target error. The analysis and bounds depend on a certain nonlinear margin quantity due to Nitanda and Suzuki, and can lead to tight upper and lower sample complexity bounds. Joint work with Ziwei Ji.
Date & Time
March 26, 2020 | 12:00pm – 1:30pm
Location
https://illinois.zoom.us/j/741628827Speakers
Matus Telgarsky
Affiliation
University of Illinois