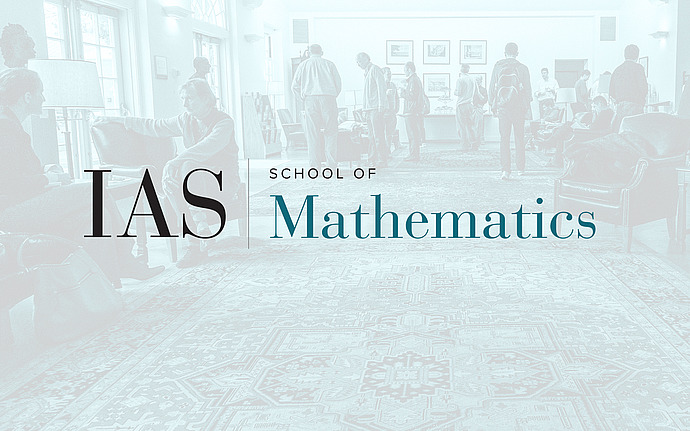
Theoretical Machine Learning Seminar
Learning in Non-convex Games with an Optimization Oracle.
We consider adversarial online learning in a non-convex setting under the assumption that the learner has an access to an offline optimization oracle. In the most general unstructured setting of prediction with expert advice, Hazan and Koren (2016) established anexponential gap demonstrating that online learning can be significantly harder. Interestingly, this gap is eliminated once we assume a convex structure. A natural question which arises is whether the convexityassumption can be dropped. In this work we answer this question in the affirmative. Namely, we show that online learning is computationally equivalent to statistical learning in the Lipschitz-bounded setting.Notably, most deep neural networks satisfy these assumptions. We prove this result by adapting the ubiquitous Follow-The-Perturbed-Leaderparadigm of Kalai and Vempala (2004).