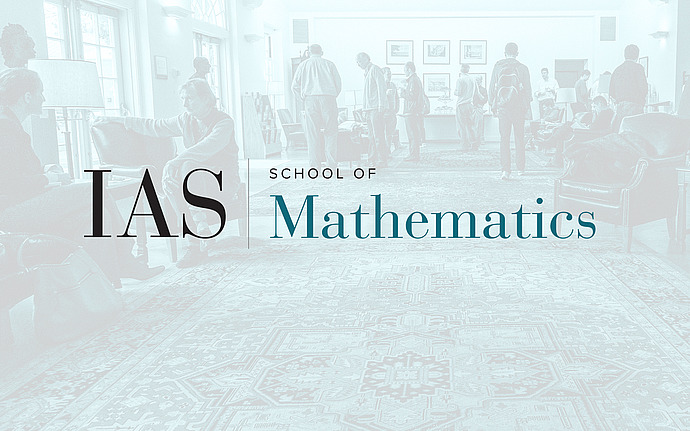
Non-equilibrium Dynamics and Random Matrices
Geometry of metrics and measure concentration in abstract ergodic theory
Many of the major results of modern ergodic theory can be understood in terms of a sequence of finite metric measure spaces constructed from the marginal distributions of a shift-invariant process. Most simply, the growth rate of their covering numbers gives the entropy of the process, and then one finds that more refined geometric invariants determine other properties of the process. Perhaps the most important among these is a characterization of processes that are isomorphic to Bernoulli (i.i.d.) processes: they are those for which these metrics exhibit a certain concentration of measure. This is easily seen to be equivalent to older characterizations of Bernoullicity due to Ornstein. Using his characterizations, Ornstein and others were also able to provide examples of `Kolmogorov automorphisms' (processes for which the distant past determines nothing) that are not Bernoulli, answering a long-standing open question. In particular, Kalikow showed that a natural family of examples describing random walks in random sceneries are of this kind. However, it remained open whether the family of examples studied by Kalikow were all distinct, or whether they really constitute only one example. Recent work has now been able to distinguish them using another new invariant, also defined in terms of the asymptotic geometry of those metric measure spaces. This talk will be an overview of this metric-measure-space approach to entropy and Ornstein theory, finishing with a sketch of the new ideas that go into the recent classification result for the examples studied by Kalikow.