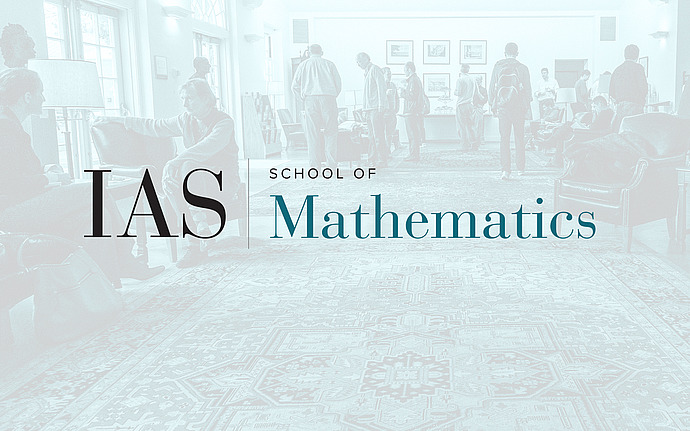
Members’ Seminar
Estimating the mean of a real valued distribution
I revisit the basic statistical problem of estimating the mean of a real-valued distribution. I will introduce an estimator with the guarantee that "our estimator, on *any* distribution, is as accurate as the sample mean is for the Gaussian distribution of matching variance." Crucially, in contrast to prior works, our estimator does not require prior knowledge of the variance, and works across the entire gamut of distributions with finite variance, including those without any higher moments. Parameterized by the sample size $n$, the failure probability $\delta$, and the variance $\sigma^2$, our estimator is accurate to within $\sigma\cdot(1+o(1))\sqrt{\frac{2\log\frac{1}{\delta}}{n}}$, which is tight up to the $1+o(1)$ factor. I will contrast these results with ongoing efforts to adapt these techniques to higher dimensions.
Based on joint work with Jasper Lee - https://arxiv.org/abs/2011.08384