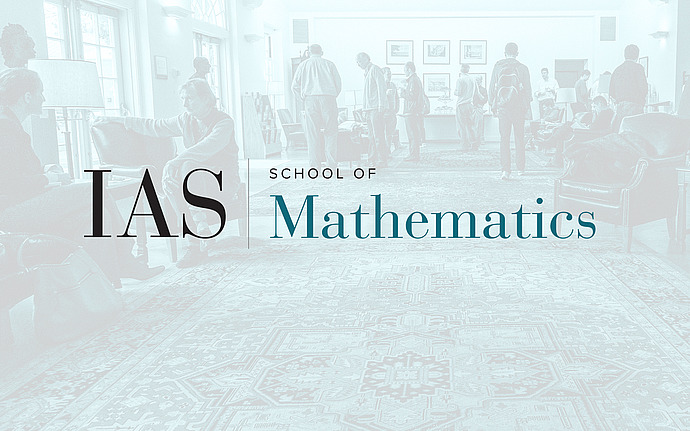
Members’ Seminar
Eigenvalue bounds on sums of random matrices
For certain applications of linear algebra, it is useful to understand the distribution of the largest eigenvalue of a finite sum of discrete random matrices. One of the useful tools in this area is the "Matrix Chernoff" bound which gives tight concentration around the largest eigenvalue of the expectation. In some situations, one can get better bounds by showing that the sum behaves (in some rough way) like one would expect from Gaussian random matrices. I will discuss recent work in understanding and exploiting such situations; this will include include interesting connections to beta ensembles and free probability.
Date & Time
November 14, 2016 | 1:15pm – 2:15pm
Location
S-101Speakers
Affiliation
Princeton University; Member, School of Mathematics