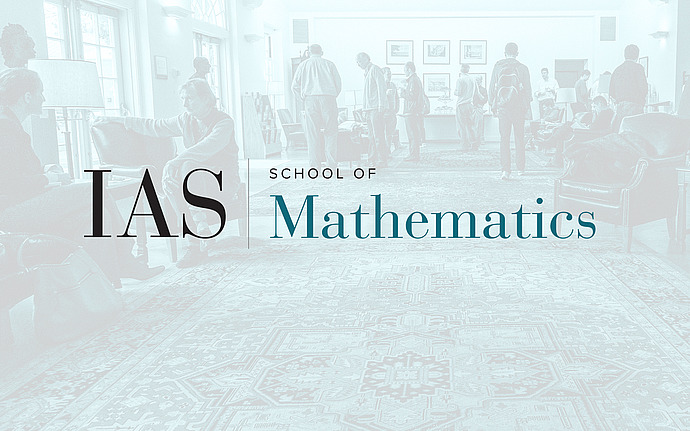
Computer Science/Discrete Mathematics Seminar II
Outlier-Robust Estimation via Sum-of-Squares
We develop efficient algorithms for estimating low-degree moments of unknown distributions in the presence of adversarial outliers. The guarantees of our algorithms improve in many cases significantly over the best previous ones, obtained in recent works. We also show that the guarantees of our algorithms match information-theoretic lower-bounds for the class of distributions we consider. These better guarantees allow us to give improved algorithms for independent component analysis and learning mixtures of Gaussians in the presence of outliers.
Our algorithms are based on a standard sum-of-squares relaxation of the following conceptually-simple optimization problem: Among all distributions whose moments are bounded in the same way as for the unknown distribution, find the one that is closest in statistical distance to the empirical distribution of the adversarially-corrupted sample.