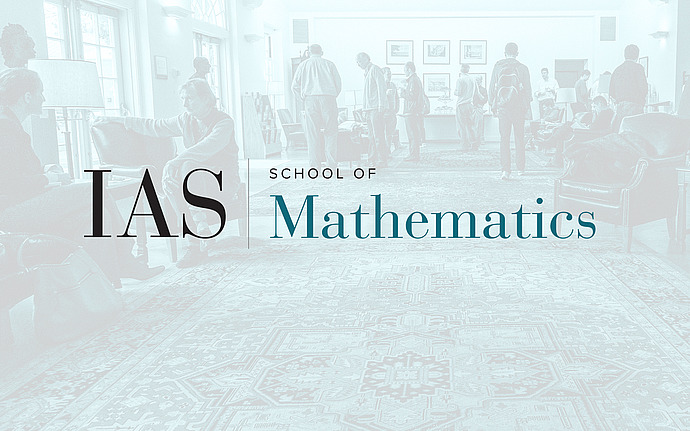
Computer Science/Discrete Mathematics Seminar II
Explicit, Epsilon-Balanced Codes Close to the Gilbert-Varshamov Bound
I will show an explicit construction of a binary error correcting code with relative distance $\frac{1-\epsilon}{2}$ and relative rate $\epsilon^{2+o(1)}$. This comes close to the Gilbert-Varshamov bound that shows such codes with rate $\epsilon^2$ exist, and theLP lower bound that shows rate $\frac{\epsilon^2}{\log \frac{1}{\epsilon}}$ is necessary. Previous explicit constructions had rate about$\epsilon^3$, and this is the first explicit construction to get that close to the Gilbert-Varshamov bound.This talk will have two parts, on Monday and Tuesday.I plan to use the first talk to explain the problem and previous solutions, and overview of the new approach. In the second talk I will discuss technical aspects, including the wide zig-zag product and how (and why) it gives a better parity sampler, explained below.Technically, we define Parity Samplers. A parity sampler is a collection of sets $\{S_i \subset \Lambda \}$ with the property that for every "test" $A \subset \Lambda$ of a given constant density $\epsilon_0$, the probability a set $S_i$ from the collection falls into the test set $A$ an \emph{even} number of times is about half. A sparse parity sampler immediately implies a good code with distance close to $\frac{1}{2}$. The complete $t$-complex of all sequences of cardinality $t$ is a good parity sampler, but with too many sets in the collection. Rozenman and Wigderson, and independently Alon, used random walks over expanders to explicitly construct sparse parity samplers, and their construction implies explicit codes with relative rate $\epsilon^4$.I will show how one can get better explicit parity samplers (and therefore also better explicit codes) using a variant of the zig-zag product. In the random walk sampler, there exist many sets with substantial overlap. One way to look at the zig-zag product is that it takes a sub-collection of the random walk sampler, and this sub-collection has a smaller overlap between sets in the collection. The zig-zag product achieves that by keeping a small internal state. I will show that by enlarging the internal state one can further reduce the overlap, and as a result improve the quality of the parity sampler.