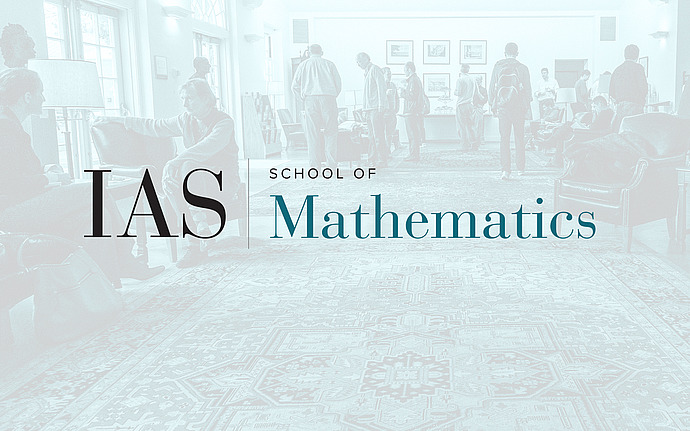
Computer Science/Discrete Mathematics Seminar II
How to round subspaces: a new spectral clustering algorithm
Given a $k$-dimensional linear subspace, consider the problem of approximating it (with respect to the spectral norm) in terms of another subspace spanned by the indicators of a $k$-partition of the coordinates. This is known as the spectral clustering problem, first introduced by [Kannan, Kumar]. It is a generalization of the standard $k$-means problem, which corresponds to the Frobenius norm. In this talk, I will present a new spectral clustering algorithm. Our algorithm finds a partition with spectral norm $O(\sqrt{OPT})$, where $OPT$ is best possible: Previously, no $o(k OPT)$ algorithm was known. I will also talk about various applications; such as decomposing a graph into disjoint union of expanders, finding a partition which minimizes the maximum expansion and so on.