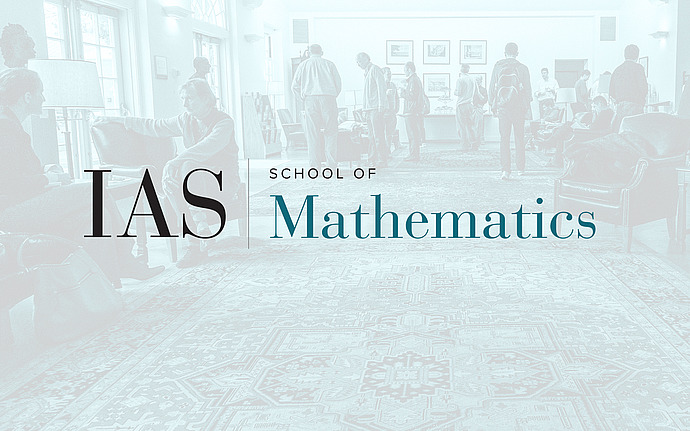
Computer Science/Discrete Mathematics Seminar I
A Complexity-Theoretic Perspective on Fairness
Algorithms make predictions about people constantly. The spread of such prediction systems has raised concerns that algorithms may exhibit unfair discrimination, especially against individuals from minority groups. While it's easy to speculate on the risks of unfair prediction, devising an effective definition of algorithmic fairness is challenging. In this talk, we will give a high-level introduction to one prominent notion from the algorithmic fairness toolbox, called multi-calibration. Multi-calibration requires that algorithmic predictions be well-calibrated (unbiased), not simply overall, but simultaneously over a rich collection of subpopulations. This ``multi-group'' notion strengthens the guarantees of group fairness definitions, without incurring the cost (statistical and computational) associated with individual protections. Our focus will be on the definition of multi-calibration, motivating it as an effective strategy for fairness in prediction, as well as highlighting connections to other concepts in learning theory and pseudorandomness.
The talk will assume no prior knowledge on algorithmic fairness, aiming to be accessible to a broad theory audience.